Accelerating UN Sustainable Development Goals with AI-Driven Technologies: A Systematic Literature Review of Women’s Healthcare
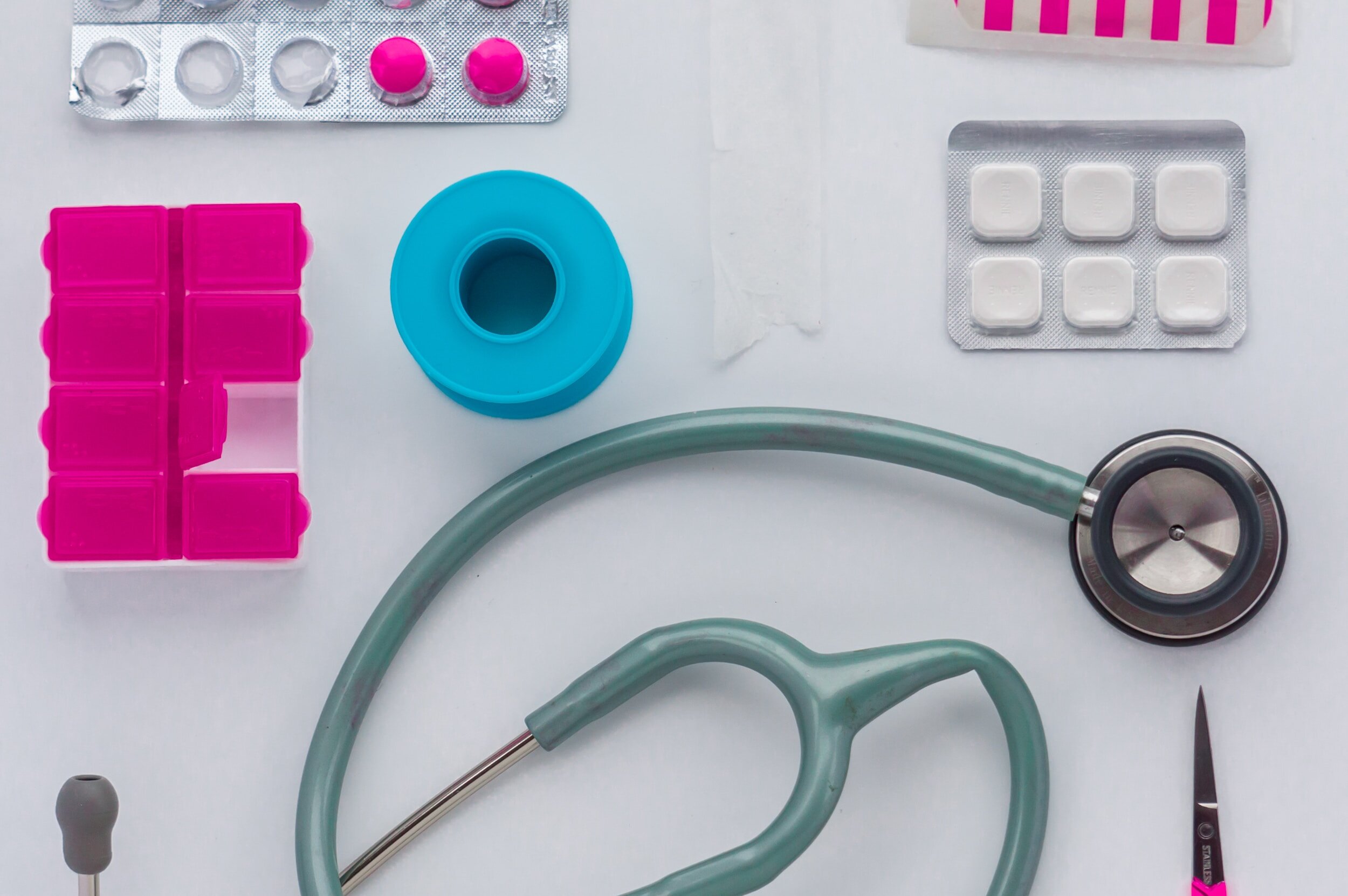
IIn a recent study on AI-driven technologies and women’s healthcare, I conducted a systematic literature review to determine whether AI-driven technologies adequately represented medical data and research into women’s healthcare. One key finding from this research was that there was clearly a lack of representative data on women’s healthcare conditions. My co-investigators and I divided the research inquiry into five thematic clusters, comprising, amongst others, gender equality and health AI. It is unsurprising that the findings of this study point to the need for a much more significant body of required research for the improvement of AI risk prediction models for many aspects of women’s healthcare. In addition, the authors note that the representation of women’s healthcare conditions are low and uneven in comparison to other available general health data, and even if sparsely available, this representation has been limited to disease-specific interventions. Hence, when AI-driven technologies or systems are deployed in medicine and healthcare, based on the limited availability of good quality and representative data regarding women, any resulting outcomes will clearly be skewed.
For example, because women have been excluded from many clinical trials, this may result in incomplete data sets to train systems for disease and illness suffered by women. Algorithmic biases present themselves vis-à-vis false diagnosis, and if this false diagnosis is relied upon, wrong treatment protocols can further exacerbate the situation. Deploying AI-driven technologies that do not account properly for differences and diversity means we are ultimately releasing into healthcare delivery, high level risks of lack of safety and inadequacy. Therefore, what is clear is that even ‘good’ algorithms (algorithms intended to be used for altruistic and noble reasons) can become sexist in their outcomes, based upon the kind and quality of data that is used to train the AI systems.